"Making an AI-designed life form would be pretty fun"
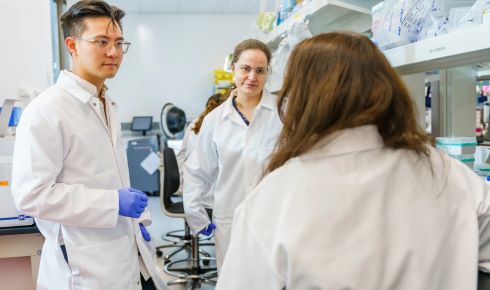
6th December 2024
AI and gene-editing pioneer Patrick Hsu talks to The Biologist about Evo – arguably the most powerful AI model in biology – and his ambitions to create a fully virtual cell
Patrick Hsu is co-founder and a core investigator at the Arc Institute, a non-profit institution for biomedical science and technology headquartered in Palo Alto, California, US. Hsu’s lab works at the intersection of biology, engineering and AI, inventing new biotechnologies and tools to accelerate scientific progress in improving human health.
This year was a big one for Hsu and the institute, which has been operating for less than three years. In February, Arc released details of Evo, a biological ‘foundation model’ that is learning the intrinsic logic of masses of genomes. The first iteration of Evo can already perform a suite of remarkable tasks across all three major biological languages, from elucidating mechanisms and relationships between DNA, RNA and proteins to designing new gene-editing systems and even entirely new genomes.
This came just a month after a preprint revealed Hsu’s group had developed an entirely new gene-editing system based on bridge RNA: non-coding RNAs normally involved in the transposal of DNA from one part of the genome to another (‘jumping genes’).
Researchers at Arc have since validated much of the remarkable data produced by Evo – for example, showing in wet lab experiments that new CRISPR systems designed by the model do actually work. Their bridge RNA system has also been shown to be capable of making insertions, excisions and inversions of DNA, suggesting it can be used for whole-scale genome redesign.
The wider context
Understanding how a model such as Evo works involves a blizzard of terminology that can be impenetrable to those without computing expertise. From an office at Arc, Hsu tells me it is an “autoregressive transformer”, which means that, like ChatGPT, its core function is simply to predict what comes next in a sequence (ChatGPT does this with words, over and over, to build up passages of plausible text in response to prompts or questions; Evo does it with nucleotides). It’s also a “long context hybrid state space model”, which Hsu admits is a lot of AI buzzwords. The key part is ‘long context’.
What has generated excitement about Evo and its ‘long context’ intelligence is that it can perform a range of different tasks just as well as – and sometimes better than – AI-bio models trained to perform specific tasks. Researchers at Basecamp Research, a UK-based AI startup, recently wrote that Evo-type models “have the potential to design more complex proteins, with specific functions, specific expressibilities and higher success rates than many of the specific protein models… it is able to do all these fantastic things because it understands the wider context that the protein evolved in”.
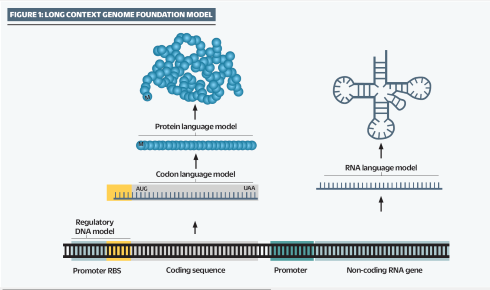
Evo can also, for example, predict the impact of mutations on fitness and function, predict gene expression from regulatory DNA, predict which genes are essential or non-essential to a genome, and more. The latter, Hsu reminds us, “is a thing people are doing real experiments to find out, which take months in the lab and cost tens of thousands of dollars”.
Hsu says: “Long context is incredibly important if you want to read across, say, an entire legal document or a series of books or, indeed, an entire genome. DNA encodes everything that you need to make a whole organism. It has all the regulatory information, it has all of the non-coding RNA, the CPG islands, promoters and the mRNAs for the proteins, which all interact in order to create molecular biology and function. Evo is able to look across all of these different elements that are embedded within DNA. So we showed that the model is not just a DNA language model, it’s a regulatory DNA language model; it’s also an RNA language model and a protein language model.”
One of its most stunning abilities is designing complex molecular systems such as CRISPR, which is made up of multiple interacting elements including genes, non-coding RNAs and proteins.
“Models such as AlphaFold can’t natively understand these things,” says Hsu. “What we showed is that the model understands the co-evolutionary relationships between these elements, which has enabled us to design new types of CRISPR systems.”
A cut above
Some describe what Evo is doing when it is generating new sequences as ‘hallucinating’, and I suggest to Hsu that imagination is a sort of informed hallucination. Is it fair to say the system is ‘imagining’ genetic sequences that would have high evolutionary fitness?
“Exactly,” he says. “The gap between ‘hallucination’ and ‘design’, to me, is basically, do they actually work? Can they actually cut DNA?”
Hsu’s latest paper, which made the cover of Science in November, shows that novel CRISPR systems designed by Evo do indeed cut DNA as effectively as state-of-the-art CRISPR tools, and Hsu says there is “preliminary evidence” that they will actually be better than those found in nature. “That implies something, I think, very provocative, which is that the sequences we mine from genomic sequencing databases are not the most evolutionarily or functionally fit,” he says.
Evo’s design work is different to ‘directed evolution’ methods for improving the functionality of biomolecules, says Hsu, which can only make incremental improvements on an existing structure.
“Our model can actually very powerfully understand secondary, tertiary and quaternary structure at massive scale in this representation space, and just design an RNA that’s optimal for the enzyme. Which I find kind of mind-blowing.”
While the development of new gene editing tools has obvious applications, Hsu is also excited about Evo’s capabilities at the whole genome scale. “We have generated genome-scale sequences over a megabase long (a million bases of DNA). We’re able to generate all of the tRNAs, rRNAs and marker genes that support protein translation and expression – these look like real genomes. So one thing we’re excited about doing in the future is to actually synthesise one of these and make an AI-designed life form. I think that would be pretty fun.”
Evo is trained on prokaryotic genomes; developing an equivalent model based on eukaryotic genomes, which are much, much, larger, will require far more computing power. Hsu describes himself as a human geneticist originally and his long-term goal is to create a ‘virtual cell’ that can help us understand and treat disease.
The aim is not simply to map everything contained in a living cell and what it does. “A virtual cell model should be able to understand dynamic cell states,” he says. “What you really want to be able to do, conceptually, is to be able to click and drag cells from cell state A to cell state B, from inflamed to normal or, from, say, mitotic to post-mitotic. That’s what we actually care about, right? Drugs are like our primitive version of click and drag. What you want is a model that can tell you the exact, multiplex perturbations and the temporal cascade that would be required in order to move between these subtypes of cell. We’re placing a big bet that a virtual cell model will help us do that.”
Virtual cell 1.0
The Arc Institute is a hybrid of wet and dry lab research, and the wet lab side is busy producing vast quantities of RNA sequencing data to train the virtual cell models. Hsu is unable to put a timeline on when a virtual cell 1.0 might be ready, but he believes that ultimately it will solve “a grand challenge in biology”.
Developing tools that will be widely used by other researchers is central to the Arc Institute’s mission to “accelerate scientific progress, understand the root causes of disease, and narrow the gap between discoveries and impact on patients”. Its tools portal has a growing list of free computational tools developed by Arc scientists, along with supporting publications, documentation and code.
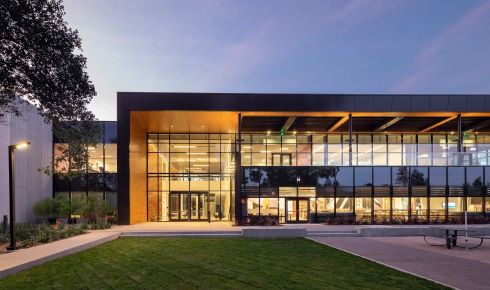
The institute is independent, but operates in collaboration with Stanford University; the University of California, Berkeley; and the University of California, San Francisco. It has a staff of traditional academic investigators and a separate R&D staff who are more akin to the workforce of a frontier AI lab or biotech company. The core investigators focus on five science fields: cell biology, neuroscience, human genetics, technology development and chemical biology. Five technology centres, focused on computing, cell models, mammalian models, gene engineering and multi-omics, aim to develop the institute’s data and scientific insights into new technologies and tools.
As we begin to wrap up our conversation, there are a couple more questions I feel the need to ask. Having been hopelessly lost at several points when the conversation turned to computing, I wonder whether it is important for biologists to understand the models they are using. Hsu – who admits that Arc researchers don’t fully understand how Evo generates the answers that it does – isn’t sure it’s necessary. “Biology runs on kits and assays and models of tools. You’ve always had that, and in computing too. Students today who work in software engineering can’t necessarily write a kernel [the program at the heart of a computer’s operating system]. Progress happens over time when you have abstractions that allow us to kind of work at higher levels of function – as long as the underlying principles are robust and determined.”
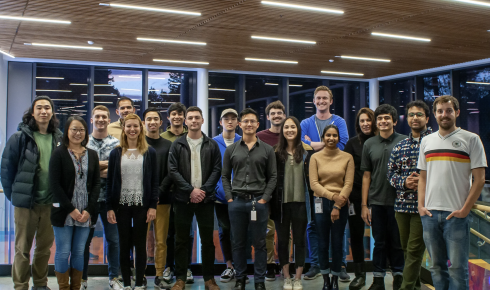
He doesn’t buy concerns about AIs being ‘black boxes’ that can’t be trusted. “I personally never really understood this scepticism, because the models that people build that are open box and interpretable often don’t model the biology very well. So it’s always made much more logical sense to me to start with a model that very faithfully models what actually happens, and then back out mechanistic principles or interpretability from there.”
Existential risk
The final issue to discuss is safety. Is Hsu worried his work could one day contribute to the development of a bioweapon or some other as-yet-unseen catastrophe?
“There’s been tremendous debates about AI safety and existential risk,” says Hsu. “I think folks get too excited. Two years ago there was a lot of AI doomerism – people saying that around now, or next year, the world is going to fall apart, that we’re going to have Skynet [the artificial super-intelligence from the Terminator movies that is at war with humanity]. You know OpenAI initially didn’t want to release ChatGPT-2 because it thought it was too powerful? And yet we’re now on GPT-4.”
Safety precautions were taken when training Evo: researchers excluded viruses from the training data that were known to infect eukaryotic hosts, for example. “We’ve ‘red teamed’ these models to align them with human preference, so that they can’t generate certain types of sequences. But how you do that really consistently and reliably is hard. These are big, complex models. There are entire companies that are working on AI safety and I do think we’ll have to do similar things for biological AI models.”
Hsu ends our conversation with an optimistic take for those who worry about the growing power of AI biology. “I think these models will help defence more than they help offence,” he says. “Offence [i.e. creating a dangerous pathogen] is relatively easy – it’s part of normal virology and gain-of-function research. It’s not obvious to me that AI massively lowers the threshold of how easy it is to do these things. But what AI can do is help us predict the likely evolution of a virus to resist a vaccine or therapy, or help us design a broadly neutralising type of defence.”
And so, Hsu’s AI models look set to continue to grow in power and scale, building their understanding of the complexities and possibilities of living systems to levels no scientist could ever comprehend.
“I think Evo 1 is learning a blurry picture of life. And as we sharpen the focus knob with more data and more scale in the future, I think a lot of systems complexity can emerge from the model. In ways that will be incredibly surprising.”
Patrick Hsu is co-founder and a core investigator at the Arc Institute