Regulating revolutions
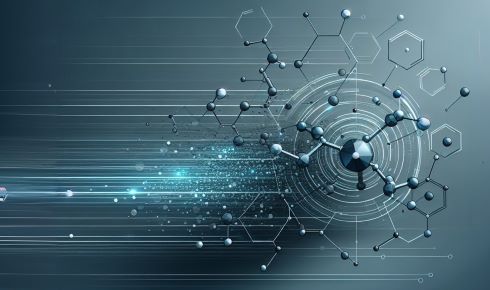
6th December 2024
As biotechnology and AI converge and advance at high speed, developing robust oversight is challenging – but not impossible, writes Sana Zakaria
The use of machine learning and AI to complement areas of life science such as biotechnology, engineering biology and synthetic biology has become commonplace in just a few years. As shown in our latest special issue, AI-powered biological design tools now provide a range of capabilities to biologists, including viral vector design, deductions of genome-phenome associations, predictions of protein structure and binding, and even the generation of entirely new proteins and genomes. In short, AI is addressing some of the biggest questions in biology, and powering innovative applications across life sciences R&D, agriculture, sustainability, pollution control, energy security, human health and defence.
Despite this rapid progress, the convergence of AI with bioscience is still in its infancy and proactive policy is needed to manage these technologies as they mature. The technology is advancing far faster than associated policy frameworks, and there is little governance specifically focused on the intersection of AI and life science.
Shifting biothreats
We are also in an era of unprecedented ‘technological diffusion’ – meaning the new biological knowledge, skills and technical capabilities spread quickly across actors and economies. New gene-editing tools, benchtop DNA and peptide synthesisers, lab-on-a-chip technologies and automated ‘cloud labs’ are enabling novel experiments to be performed at scale, in silico, sometimes even without the need for much biology or coding experience. This diffusion, combined with ease of access to sophisticated biological design tools, open science papers and biological data sets, machine learning codes and other biotechnologies, is rapidly changing who can do bioscience, and what they can do, shifting the risk landscape of a range of biothreats.
While this suite of technological innovations offers great promise in tackling some of our most pressing challenges – from new treatments for diseases to mitigation of environmental threats – it is not a big leap to consider how many of these tools and capabilities could be used for harm. An eye-opening proof of principle was demonstrated in 2022 when researchers conducted an impromptu experiment using a biological design tool, originally intended to screen for drug candidate toxicity, which was able to generate the details of 40,000 toxins, both known and novel, without undergoing training to do so¹.
The most commonly discussed risk scenarios associated with AI and bioscience are concerned with the generation and synthesis of new synthetic pathogens with the potential to enable pandemic-level catastrophes. Autonomous AI agents could be used to engage with remote-operated cloud laboratories to conduct dangerous experiments, or large language models could provide instruction on how to create harmful genetic sequences.
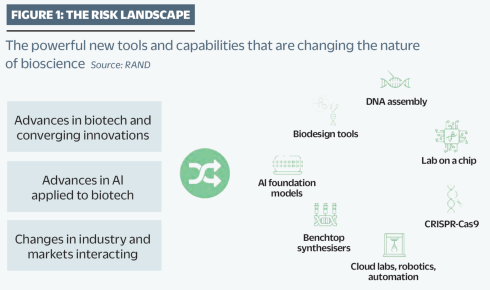
Dangerous pathogens can, of course, be cultivated and released without access to sophisticated AI or machine learning tools – various individuals and organisations have deployed biological agents such as Bacillus anthracis (anthrax) or botulinum and ricin toxins over the last 50 years, and more primitive biological warfare goes back many centuries.
Elsewhere in our most recent issue, AI pioneer Patrick Hsu argues that AI-powered bioscience could prove to be more useful “as defence rather than offence” – for example, helping scientists to predict the evolution of a new pathogen or bioweapon and find a suitable antidote or vaccine.
However, understanding how and when risks may emerge is a critical area for policymakers, and a comprehensive characterisation of risks is warranted. Understanding the potential pathways to the development of harmful biological agents, and the kind of capabilities, tools and resources needed to put a harmful use into action, is the starting point for a meaningful assessment of risk, which can then inform various governance approaches.
Stress tests
Any governance of these technologies must balance the risks with the vast potential for societal benefit – we want to avoid stifling innovation that could improve lives. The formulation of policies depends on both the acceptability of trade-offs and level of risk to policymakers and the broader public.
A variety of assessment frameworks and scenarios are being used to characterise and stress test the feasibility and likelihood of certain risks occurring at a given point in time. For instance, researchers at RAND² and ChatGPT developer OpenAI³ separately set out to assess the hypothesis that large language models could be used to provide additional knowledge to weaponise biological data sets. Both studies actually concluded that there is no statistically significant added value to using the models at present, but the results indicate that on certain parameters, such as accuracy and completeness of tasks, there could be an advantage over not using the large language models. However, these language models have since evolved, with newer and more powerful versions such as ChatGPT-4 now available publicly, warranting renewed (and perhaps continuous) assessment.
Other scenarios and hypotheses being assessed include the use of biological design tools to create pathogenic variants of known diseases; the use of language models and design tools to enable a non-expert to design a new pathogenic agent; and the use of commercial companies to produce toxin sequences on behalf of a bad actor, which could be hidden from biosafety systems by being broken up into smaller sequences, before being assembled in a remote-operated wet lab environment. Analysis involves understanding the possible capabilities of the technological tools, but also the level of expertise and skills required, and the pathways or sequence of events that could lead to catastrophic outcomes.
Keeping pace
Oversight and governance at the intersection of technologies is inherently complex, but it is even more so given the fast pace of technological progress at the moment. AI and machine learning, and synthetic and engineering biology, are advancing at extraordinary speeds, often – but not always – separately.
AI presents an interesting case for evaluating whether hard law mechanisms, such as the recent EU AI Act, can ever work as well and be as agile as adaptive and non-voluntary mechanisms. History has shown that legally enforceable or ‘hard law’ mechanisms can be extremely challenging to change in step with fast-moving technological progress, with the EU’s directive on genetically modified organisms being a clear example. This legislation has effectively prevented any genetic changes to crops and animals intended for human consumption in the EU for years, even as genetic techniques have changed drastically.
Similar cases can be found in laws governing human embryology, which have struggled to remain relevant in the face of breakthroughs such as stem-cell-based embryo model systems (SCBEMs). In this case, adaptive and ad hoc mechanisms, such as the UK-led code of practice for SCBEMs, have been developed for use as a stop gap to the lack of legally enforceable mechanisms in place.
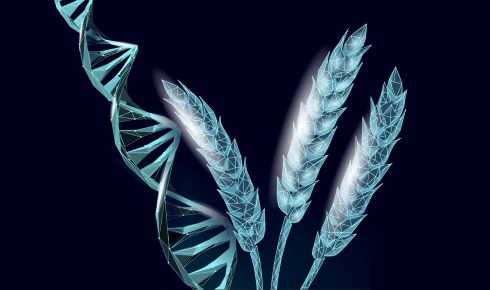
Similar ad hoc and non-legally-binding mechanisms are emerging for both general AI and for biological tools, such as the US-led executive order on AI4 and a framework for safe protein design⁵ proposed by a group of academics and clinicians earlier this year. However, the current patchwork of policy interventions and oversight mechanisms make cooperation and enforcement of standards and safety at a global level challenging.
Even in the patchwork, a range of gaps, loopholes and exceptions exists. For example, although the US’s AI Executive Order has established reporting requirements for the AI industry that includes biological design tools, reporting is only required if models and tools use a certain computational power threshold; many powerful tools operate under that threshold, including Nobel Prize-winning AlphaFold⁶. The EU’s AI Act only makes references to general-purpose AI (such as ChatGPT) and the potential for them to be used in bioweaponisation, but does not extend to the many biological design tools now available.
Another challenge comes from the nucleic acid synthesis sector. Commercial companies are not mandated to screen for potentially harmful sequences in their orders from customers and there are no internationally mandated standards for protocols on screening, with policies and safeguards differing between companies and countries. However, the work being done by the International Gene Synthesis Consortium has led to some consistency and cohesion in screening in industry and academia, and the UK Government has very recently released guidance⁷ in its ‘baseline expectations’ for screening synthetic nucleic acids, which are positive developments.
National to international
The UK has no specific oversight for biological design tools, but has established the AI Safety Institute, which will research and develop governance and policies, and the infrastructure required to test the safety of AI models. A holistic approach to oversight, spanning the pillars of the UK Biological Security Strategy – ‘understand, detect, protect and respond’ – is a useful way of assessing risks and responding to them with various policy interventions.
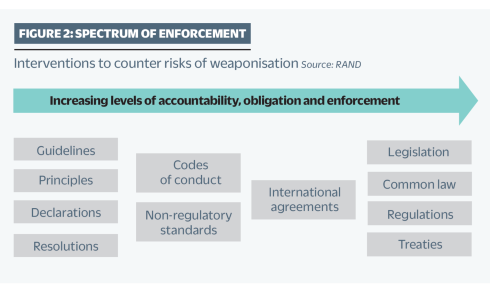
This involves undertaking research to understand and categorise risks, including the risks of certain data sets, and ‘red teaming’ tools before their release. Red teaming is a simulated attack, where an independent group or ‘red team’ attempts to breach an organisation’s security with the aim of highlighting the impact of a successful attack and what works to defend against it. Embedding a diversity of perspectives, with deep understanding of the risks, the domain, and the actors or adversaries, is essential to improve a red team’s effectiveness. There are other mechanisms that complement the red-teaming approach.
Enforceable guidelines and screening regimes, such as global surveillance and microbial forensics, can then further deter malicious or nefarious uses, and investment in surveillance and preparation of the necessary medical countermeasures can help ensure an effective response if worst-case scenarios ever come to pass.
It is likely that a suite of interventions across a spectrum of enforcement – from sector-led guidelines to international treaties – is needed to assess and mitigate against risks of weaponisation (see figure 2, above).
Who will do this difficult work? Industry, academia and government all have a role to play in strengthening the science-policy interface where governance will develop. Public engagement and perception are crucial to consider in future policymaking, and there is also the culture gap between the machine learning/AI communities and biologists to try to bridge.
Industries, especially large AI labs, have become critical to the policy debate and have an important role to play in supporting policy development, holding themselves accountable and operating within a level of risk that is acceptable. Academics are also in a unique position, as many straddle both academic research and commercial enterprises, and could be well suited to devising risk management approaches and policy interventions.
Similarly, scientists and academics have a critical role to play in engaging policymakers with the science underpinning the risks and the policy ramifications of their research, helping identify hazards and potential risks in their fields as early as possible.
International alignment
Given the exciting opportunities as well as the risks that arise from the convergence of these maturing technologies, international cooperation and alignment have never been more important. Not securing international standards and safety measures for emerging technology oversight and its deployment could be catastrophic for global biosecurity.
Instruments such as the Biological Weapons Convention and WHO International Health Regulations should be utilised further to aid in gaining consensus on these issues and how they can be mitigated with cohesive international policies. The time to act on these is now, as the rapidity at which technology is evolving is set to continue.
Dr Sana Zakaria FRSB is a research leader and Global Scholar at RAND, a non-profit institution that helps improve policy and decision-making through research and analysis. She is currently leading RAND Europe's Biotechnology policy research focusing on biosecurity and pandemic preparedness.
1) Urbina, F. et al. Dual use of artificial-intelligence-powered drug discovery. Nat. Mach.
Intell. 4, 189–191 (2022).
2) Mouton, C. et al. The operational risks of AI in large-scale biological attacks. RAND,
16 October 2023.
3) Building an early warning system for LLM-aided biological threat creation. OpenAI, 31 January 2024.
4) Executive order on the Safe, Secure and Trustworthy Development and Use of Artificial Intelligence. The White House, 30 October 2023.
5) Community Values, Guiding Principles, and Commitments for the Responsible Development of AI for Protein Design. responsiblebiodesign.ai
6) Managing Risks from AI-Enabled Biological Tools. Centre for Governance on AI. 5 August 2024.
7) UK screening guidance on synthetic nucleic acids for users and providers. Department for Science, Innovation and Technology. Published 8 October 2024.